Strain rate, size and defect density interdependence on the deformation of 3D printed microparticles
Statistical significance in materials science is a challenge that has been trying to overcome by miniaturization. However, this process is still limited to 4-5 tests per parameter variance, i.e. Size, orientation, grain size, composition, etc. as the process of fabricating pillars and testing has to be done one by one. With this project, we aim to fabricate arrays of well-defined and located particles that can be tested in an automated manner. With a statistically significant amount of samples tested per parameter variance, we expect to apply more complex statistical models and implement machine learning techniques to analyze this complex problem.
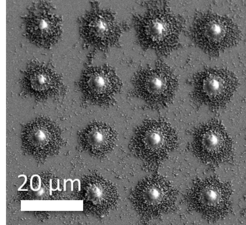
The mechanical metrology of micro/nano-scale metallic systems has critical implications for both scientific and industrial communities. However, there are very few studies that explore the mechanical behavior at small- scales under such extreme conditions in a statistically relevant manner. Through this project, we aim at tackling this critical lack of understanding in the mechanical behavior of small-scale metals. To that end, additively manufactured metal microparticles will be used to investigate the effect of size, defect density, strain rate and temperature on their compressive behavior in an automated manner. Subsequently, we will perform annealing of these particles to tackle the grain size and hence the defects in the samples. Further, in situ compression tests on these different microparticle arrays will be used to investigate the effects of strain rate and temperature interdependence. These tests will be conducted in an automated manner. In addition, microstructural characterization using TEM+EBSD analysis will be performed prior to and post-test to reveal the deformation mechanisms responsible for the mechanical behavior at such extreme loading conditions. Concurrently, this experimental dataset in combination with already existing constitutive laws will be fed into a machine learning pipeline. Here the objective is to identify the key parameters among initial sample conditions and mechanical loading conditions that significantly influence the mechanical behavior of microscale metals.