Software for quantitative three-dimensional imaging of short/long-range order
Here, we aim to develop machine-learning enhanced atom probe tomography approaches to reveal chemical short/long-range order (S/LRO) in a series of metallic materials.
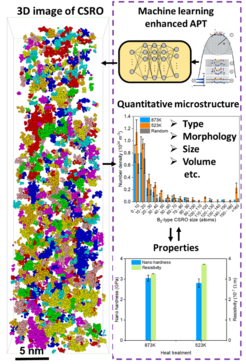
Ordering refers to atoms of specific elements self-organising within a disordered crystalline matrix. These particular atomic neighbourhoods across different length-scales can modify the mechanical and functional performances of materials. Quantitative assessment of ordering, especially SRO, in three-dimensions remains extremely challenging. Here, we used artificial neural networks to break the inherent resolution limits of atom probe tomography and reveal three-dimensional analytical imaging of the size and morphology of multiple S/LRO.
We showcase our approach by addressing a series of long-standing questions, like, encountered in a body-centred-cubic Fe-18Al (at.%) solid solution alloy that sees anomalous property changes upon heat treatment. The proposed strategy can be generally employed to investigate other ordering phenomena in a vast array of materials and help design future high-performance materials.
The software is available at GitHub address https://github.com/a356617605.