Machine Learning Potentials Including Magnetism
The Magnetic Moment Tensor Potentials (mMTPs) are a class of machine-learning interatomic potentials, which could accurately reproduce both vibrational and magnetic degrees of freedom as provided, e.g., from first-principles calculations [1]. Application to prototypical bcc iron has demonstrated that these potentials are capable to quantitatively approximate local magnetic moments, energies, and forces for various magnetic states. In this project, a number of applications such as the computation of phonon spectra in ferro- and paramagnetic states as well as molecular-dynamics simulations including spin-flips are explored.
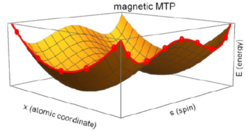
Magnetic contributions critically affect phase stability, vibrational properties, interstitial energies, local and extended defects, and kinetics. Therefore, consideration of the magnetic degrees of freedom is a prerequisite for computationally-aided design and development of a large number of technologically relevant magnetic materials.
One of the most popular computational methods, capable of capturing magnetism, are first-principles calculations realized by density functional theory (DFT) which is usually, however, computationally expensive and limited to small system sizes and to a small number of magnetic configurations. On the other hand, recent progress in machine-learning potentials has significantly accelerated DFT-based simulations for various materials. Such potentials feature near-quantum mechanical accuracy at a computational efficiency of the order of classical interatomic potentials by expressing the interatomic energy as a function of atomic positions alone. However, such potentials can usually not distinguish different magnetic states, simply because different magnetic states feature different energies and the functional form of standard machine-learning potentials typically prohibits to capture such a magnetically-induced energy variation. To overcome this fundamental shortcoming we apply in the present project the recently developed magnetic Moment Tensor Potentials (mMTPs). As opposed to the originally developed MTPs [2,3] in which the potential energy depends only on atomic positions, in mMTPs the degrees of freedom are atomic positions and spins [1].
A number of properties will be explored, e.g., computation of anharmonic free energies in paramagnetic iron or the computation of vacancy formation energies depending on the magnetic state. For this purpose, the spin-space averaging technique will be combined with molecular dynamics simulations, which combination is otherwise presently computationally hardly affordable by brute-force ab initio calculations.
Further reading
Machine learning potentials are also used, e.g., to study high entropy alloys.
Acknowledgments
This work has received funding from the Deutsche Forschungsgemeinschaft (DFG, German Research Foundation) under Project number 429582718.
References
[1] I. Novikov, B. Grabowski, F. Körmann, and A. Shapeev, Magnetic moment tensor potentials for collinear spin-polarized materials reproduce different magnetic states of bcc fe (2021), arXiv:2012.12763 [physics.atom-ph].
[2] A. V. Shapeev, Multiscale Modeling & Simulation 14, 1153 (2016).
[3] K. Gubaev, E. V. Podryabinkin, G. L. Hart, and A. V. Shapeev, Computational Materials Science 156, 148 (2019).