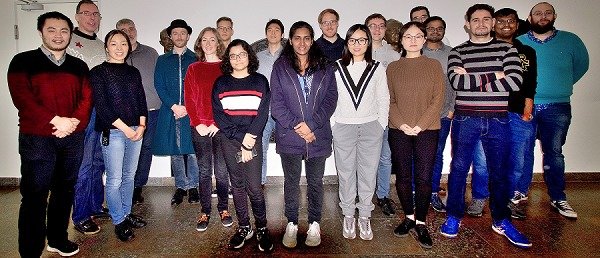
Machine-learning based data extraction from APT
Advanced microscopy and spectroscopy offer unique opportunities to study the structure, composition, and bonding state of individual atoms from within complex, engineering materials. Such information can be collected at a spatial resolution of as small as 0.1 nm with the help of aberration correction. With advancements in electron source brightness and detection efficiency, acquiring spatial maps in 2D and even 3D has become a routine practice, generating ever bigger datasets to handle.
Researchers at the MPIE now make use of tools from the big data community. An example is the analysis of the field desorption patterns obtained from atom probe tomography (APT). APT maps the 3D position and chemical identity of up to a billion atoms in a material. The pattern formed on the single-particle detector often reveal traces of the crystallographic structure and orientation of the specimen. However, it remains largely under-utilised due to the lack of efficient and accurate extraction techniques. We have proposed a mixture of feature detection, machine learning and deep learning to enable automatic identification of crystallographic patterns observed on atom probe detector maps. Preliminary results exploiting theoretical geometric relationships have been very promising. A flow-chart outlining this process is shown in the Figure below. For detector hit-maps obtained from pure-Al specimens, our approach correctly characterised the orientation of single crystals with 98% confidence. Further forays into deploying big-data methodologies to advance APT data processing is a current focus of this project.
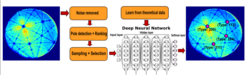