Automatic Classification and Feature Extraction from Multi-Dimensional STEM Data
In order to prepare raw data from scanning transmission electron microscopy for analysis, pattern detection algorithms are developed that allow to identify automatically higher-order feature such as crystalline grains, lattice defects, etc. from atomically resolved measurements.
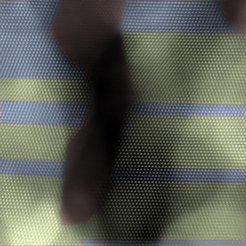
Modern scanning transmission electron microscopy (STEM) instrumentation provides not only extremely high spatial resolution at high acquisition rates (and hence monitoring dynamics in-situ), but may also characterize the sample locally via electron-energy losses. This adds spectroscopic information together with time or variations of experimental parameters as third or fourth dimension to the usual 2D image. At present, the wealth of information cannot be fully exploited due to the lack of versatile analysis tools.
In this project, we support multi-dimensional STEM analysis using unsupervised learning: crystallographic segmentation of the 2D scan plane, and feature recognition/discovery within the full data set. Spatial segmentation is necessary to identify the crystallographic and microstructural characteristics of the sample, notably the extent, identity, and lattice orientation of crystalline regions.
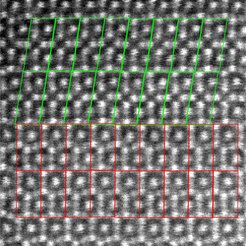
As a first success, we managed to automatically detect and differentiate different crystallographic phases based on their local periodicity from High-Angle Annular Dark Field (HAADF) micrographs. This allows for a crystallography-based segmentation of the scan plane, that can be used to improve the signal to noise ratio of the (average) unit-cell appearance of not only the HAADF channel, but all simultaneously recorded channels from other detectors.
The project is funded by and carried out within a Max-Planck wide network on big-data driven material science, called BiGmax.
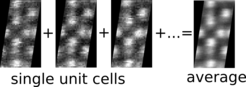