Learning Dynamics of STEM Data by Enforcing Physical Consistency with Phase-Field Models
The project’s goal is to synergize experimental phase transformations dynamics, observed via scanning transmission electron microscopy, with phase-field models that will enable us to learn the continuum description of complex material systems directly from experiment.
Scanning transmission electron microscopy (STEM) instrumentation allows one to image phase transformations at the microstructural level with a fine spatial resolution. However, a quantitative analysis of STEM video sequences is limited due to the absence of appropriate analysis tools. For instance, the spatial location of phase boundaries and their time evolution should be identified automatically despite the significant Poisson noise inherent to the imaging process. Even though in-situ STEM can image the evolution of microstructure under the influence of an external stimulus, a quantitative comparison with mesoscopic phase transformation theories remains elusive.
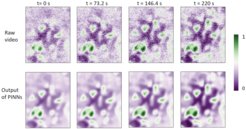
In this project, we develop machine learning approaches, particularly deep neural networks-based ones, for extracting information about the kinetics of microstructure evolution that also ensures physical consistency with phase-field models. The modelling approach can be categorized into two parts: (i) we employ physics-informed neural networks (PINN) that are trained to reproduce the STEM video sequences in a coarse-grained sense, to learn the unknown parameters of the consistent phase-field model. (ii) If the model itself is not known, then we aim at employing dictionary learning to discover a suitable phase-field model directly from data by identifying correct candidates from the dictionary. Through these approaches, we intend to build data-driven differentiable continuum models that also embrace physical significance.
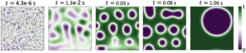
The preliminary focus is to evaluate spinodal decomposition in the AgCu system using the conservative Cahn-Hilliard phase-field model. This system allows establishing concentration-based phase-field models to directly link the experimental observations and the model based on physical parameters. Therefore, we will be able to extract bulk and interfacial free energies, the evolution of concentration fields and interface mobilities directly from STEM.
The project is funded by and carried out within a Max-Planck wide network on big-data driven material science, called BiGmax.