From electrons to phase diagrams with classical and machine learning potentials
The computational materials design department in collaboration with the Technical University Darmstadt and the Ruhr University Bochum developed a workflow to calculate phase diagrams from ab-initio. This achievement is based on the expertise in the ab-initio thermodynamics in combination with the recent advancements in machine-learned interatomic potentials and the continuous development of the pyiron workflow framework.
It enables a direct comparison of theoretically predicted phase diagrams from atomistic simulation and phase diagrams based on experimental measurements constructed with the Computer Coupling of Phase Diagrams and Thermochemistry (CALPHAD) method. While the current prototype still shows slight deviations between the theoretical and experimental phase diagrams, the workflow can be leveraged to optimize the thermodynamic approximation, analyze the dependence of thermodynamic properties on the choice of pseudo potential and compare different machine-learned interatomic potentials.
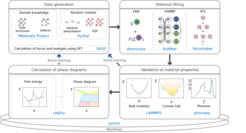
The workflow to calculate phase diagrams is divided into four steps:
- Starting with the data generation, a diverse training set is generated using the ASSYST[1] method to sample the whole phase space. The resulting structures, in combination with selected prototypes from the materials project are evaluated with Density Functional Theory (DFT).
- Afterwards, either classical or machine-learned interatomic potentials can be fitted to this dataset. At the current stage the workflow supports, classical embedded atom method (EAM) potentials and two types of machine-learned interatomic potentials the neural network potentials and the atomic cluster expansion (ACE) potentials[2].
- Following, the fitting the potentials are validated in three levels. First, by comparing the predicted energies and forces to the energies and forces calculated with DFT. Second, by comparing materials properties calculated with DFT to the materials properties predicted by the potentials and finally by calculating material properties which are not accessible by DFT and compare to experiment.
- In the current implementation, the calculation of the phase diagram is the target material property compared to experiment. The phase diagram is calculated using the CALPHY[3] package to compute the free energy differences of selected phases. While it is commonly not required, it is possible to extend the training set to further improve the predictive capabilities of the interatomic potentials using an active learning loop.
References:
- M. Poul, L. Huber†, E. Bitzek, J. Neugebauer, Phys. Rev. B 107, 104103 (2023)
- R. Drautz, Phys. Rev. B 99, 014104 (2019)
- S. Menon, Y. Lysogorskiy, J. Rogal, R. Drautz, Phys. Rev. Materials 5, 103801 (2021)