Advancing atom probe towards true atomic-scale analytical tomography
Atom probe tomography (APT) is one of the MPIE’s key experiments for understanding the interplay of chemical composition in very complex microstructures down to the level of individual atoms. In APT, a needle-shaped specimen (tip diameter ≈100nm) is prepared from the material of interest and subjected to a high voltage. Additional voltage or laser pulses trigger the evaporation of single ions from the tip.
Accelerated by the voltage, the ions fly towards a position-sensitive channel-plate detector. The time-of-flight reveals the mass-to-charge ratio from which the elemental identity of each detected ion is deduced. Datasets of up to 109 ions are routinely acquired. From a data science perspective, APT datasets are a gold mine. APT data sets are huge, noisy, and full of more or less hidden patterns.
Data evaluation involves first building a virtual 3D point cloud in which the position of each ion prior to field evaporation is reconstructed. Beyond this first step, unbiased, quantitative analysis of the information encoded in the data remains challenging. A few examples: (1) elemental identities must be correctly assigned from a potentially complex mass spectrum that contains not only atomic ions, but also often metastable small molecular units; (2) the reconstructed positions are affected by uncertainties that blur out any underlying crystalline structure; (3) microstructural features must be extracted – what phases and crystal grains do exist? Where are their boundaries? How, where, and why do compositions change?
To standardize mass spectra analysis, in particular when it comes to identify the most likely ion when multiple candidates exist for the same mass-to-charge ratio, we demonstrated that deep neural networks can be trained to disentangle mass spectra [1]. This has the potential to enormously improve the reproducibility of mapping the mass-to-charge ratio to a set of chemical identity, compared to ranges defined by human inspection which could lead to composition significant variations between experts [2].
Current APT reconstructions are based on a geometrical projection algorithm from the detector position onto a spherical cap, which largely ignores the details of the underlying physics. In reality, trajectory aberrations occur due to the local environment prior to evaporation or mesoscopic field variations associated to surface roughness. The order of evaporation is not exclusively dictated by position, but possibly also by chemical identity and bonding environment, and affects the reconstruction process causing limitations of the spatial resolution. In order to quantify these effects, the group of C. Freysoldt has started systematic theoretical investigations of field evaporation mechanisms. In addition to confirming a material-dependent tendency for roll-over at steps on faceted surfaces, they were also able to demonstrate the crossover from a two-step to a one-step mechanism controlled by the field strength [3]. It was further shown that the surface mobility of hydrogen in presence of the field and the tendency for formation of H-containing ions depend primarily on the charge transfer of the adsorbed H.
Another topic of interest is to recover the crystalline lattice structure even when the positional uncertainty of the reconstruction prevents direct identification. The crystal orientation often gives rise to a systematic intensity pattern on the detector, which can be analysed by deep-learning [4]. Grain boundaries lead to a discontinuous pattern that can be identified and tracked automatically as the data set is sliced along the evaporation order, i.e. a proxy for the depth [5]. This approach allows for direct data filtering and quantification of chemical segregation to the boundary.
Crystallographic information of APT specimen is directly accessible by using field ion microscopy (FIM). FIM is a precursor technique in which an imaging gas is continuously ionized above the surface held below evaporation threshold. A new experimental approach was initiated at the MPIE in 2018 combining FIM with enabled mass spectrometry enabled by a correlative data analysis. We used this so-called analytical FIM to directly image solute segregation to dislocations [6] and to vacancies in Ni-based binary alloys [7]. These experimental investigations were paralleled by theoretical investigations to explain the chemical contrast observed in FIM by an electronic-structure effect.
At larger scales, the formation of phases with different chemical composition is of common interest. When formed at the nanoscale, there can be dozens or even hundreds of small precipitates in a single APT data set. While they are easily visualized by concentration iso-surfaces of key elements, this is impractical to perform quantitative analysis. Therefore, the Paraprobe toolset was developed [6]. It not only allows a scriptable, well-parallelized high-throughput analysis on high-performance computers without live interaction from a human scientist, but also provides standard aggregated (e.g. radial distribution functions) and iso-surface based segmentations in an open data format (based on HDF5) that can be further processed with standard data science and visualization tools.
More recently, we have explored alternative 3D phase segmentation algorithms. They start by mapping out the chemical composition of small voxels (102-103 atoms). Despite the large scatter, clustering in composition space allows to distinguish possible phases without prior information and derive classifiers from it. Next, a DBSCAN cluster analysis in the voxelized dataset labelled according to the composition classifier successfully provides a very fast and robust composition-based segmentation. These segments can then be further analysed based on their geometrical character (e.g. line-like, plate-like, ellipsoidal, etc.) and the application-oriented scientific questions.
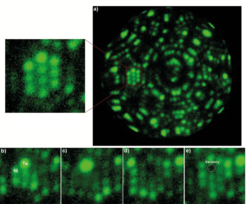
References
- Wei, Y.; Varanasi, R. S.; Schwarz, T.; Gomell, L.; Zhao, H.; Larson, D. J.; Sun, B.; Liu, G.; Chen, H.; Raabe, D.; Gault, B.: Patterns 2 (2021) 100192.
- Haley, D.; Choi, P.; Raabe, D.: Ultramicroscopy 159 (2015) 338.
- Ashton, M. W.; Mishra, A.; Neugebauer, J.; Freysoldt, C.: Phys. Rev. Lett. 124 (2020) 176801.
- Wei, Y.; Gault, B.; Varanasi, R.S.; Raabe, D.; Herbig, N.; Breen, A.J.: Ultramicroscopy 194 (2018) 15.
- Wei, Y.; Peng, Z.; Kühbach, M.; Breen, A. J.; Legros, M.; Larranaga, M.; Mompiou, F.; Gault, B.: PLoS One 19 (2019) 1.
- Morgado, F. F.; Katnagallu, S.; Freysoldt, C.; Klaes, B.; Vurpillot, F.; Neugebauer, J.; Raabe, D.; Neumeier, S.; Gault, B.; Stephenson, L.: Scr. Mater. 203 (2021) 114036.
- Kühbach, M. T.; Bajaj, P.; Zhao, H.; Çelik, M. H.; Jägle, E. A.; Gault, B.: npj Comp. Mat. 7 (2021) 21.