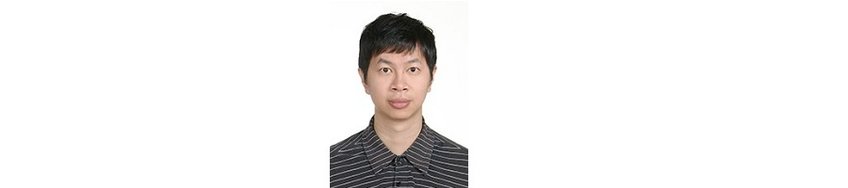
Machine Learning Enabled Materials Design: Low-Modulus Ti Alloys
A neural-network machine called “βLow” enables a high-throughput prediction for new β titanium alloys with Young’s moduli lower than 50 GPa as shown in Figure 1. This machine is trained by a very general approach with small data from experiments. Its efficiency and accuracy break the barrier for alloy discovery. The best recommendation, Ti-12Nb-12Zr-12Sn (in wt. %) alloy, from βLow is unexpected in previous methods but actually very successful. This new alloy meets the requirements for bio-compatibility, low modulus, and low cost, and holds promise for materials applied in orthopedic and prostheses implants. Moreover, the prediction of βLow indicates that the unexplored space of the chemical compositions of low-modulus biomedical titanium alloys is still large. Machine-learning-aided materials design accelerates the progress of materials development and reduces research costs in this work.
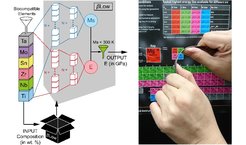
Figure 1 The schematic diagram showing that machine learning enables development of low-modulus Ti alloys
Prof. Hung-Wei Yen
Associate Professor
Department of Materials Science and Engineering
National Taiwan University
No. 1, Sec. 4, Roosevelt Road, Taipei, 10617
Taiwan(R.O.C.)
Phone | +886 2 33661335 |
Prof. Hung-Wei Yen | |
Http | Prof. Hung-Wei Yen |
Microstructure and Defects Physics Group | |
National Taiwan University |