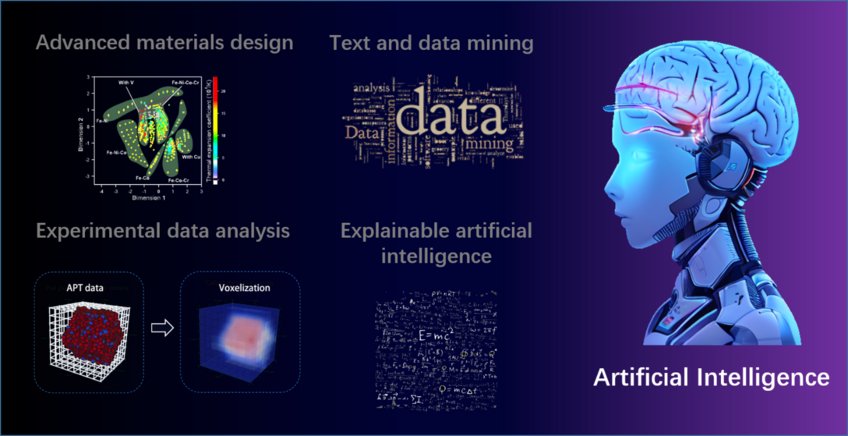
Artificial Intelligence for Materials Science
Welcome to the Artificial Intelligence for Materials Science group, where we are at the forefront of integrating cutting-edge AI technologies into the world of materials discovery and design. Our research is dedicated to transforming how materials are conceptualized, characterized, and optimized by developing next-generation AI tools and frameworks tailored to the unique challenges of materials science
Our Vision
We envision a future where AI-driven materials research not only accelerates the discovery of new materials but also ensures that these materials contribute to a more sustainable and innovative future. By leveraging the synergy between AI and materials science, we aim to drive breakthroughs in alloy design, materials discovery, and experimental data interpretation, ultimately pushing the boundaries of what’s possible in sustainable materials innovation.
Key Research Domains
2. Interpretable Physics Informed Machine Learning for Alloy Design
Alloy design is traditionally an iterative process. We focus on developing interpretable and physics-informed machine learning frameworks that bring transparency to complex predictions, making it possible for researchers to not only trust AI recommendations but also understand the scientific rationale behind them. Our work aims to develop new materials faster and more efficiently by coupling machine learning algorithms with experimental feedback loops. We have already completed two projects in this direction where the key focus has been the use of physics-informed machine learning models to predict and optimize the strength of face-centered cubic (FCC) high-entropy alloys (HEAs). We have employed a novel Residual Hybrid Learning Model (RELM), to address challenges posed by sparse, skewed datasets. By leveraging Shapley Additive Explanations (SHAP) and Partial Pair Dependencies, our machine learning model offers insights into the underlying relationships between composition, processing, and properties. This level of interpretability allows us to make informed predictions and optimizations, ensuring that the designed materials meet both theoretical and practical performance benchmarks. Another breakthrough in this study involves the integration of processing parameters such as cold rolling and annealing into our model, resulting in predictive capabilities that extend beyond theoretical compositions to actual material performance. In a separate study focused on high-entropy Invar alloys, we developed an active learning strategy that integrates machine learning with density-functional theory (DFT) and thermodynamic calculations (Figure 1). This closed-loop framework allowed us to efficiently process millions of potential alloy compositions, ultimately identifying two HEAs with exceptionally low thermal expansion coefficients. This active learning framework represents a significant advancement in automating the discovery of functional materials with optimal mechanical, magnetic, and thermal properties.
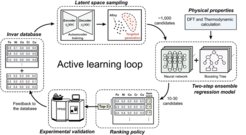
2. High-Throughput Discovery of Sustainable Materials
We are committed to the high-throughput discovery of materials that are both innovative and sustainable. Our AI models help identify materials with desirable environmental properties, such as recyclability and low energy consumption during production. Through a combination of AI-driven screening and experimental validation, we have begun exploring new categories of materials aimed at reducing the carbon footprint in industries such as steel production and lightweight alloy manufacturing. An ongoing project in this direction is harnessing advanced machine learning tools to develop high copper tolerant TRIP steels motivated from steel scrap.
3. AI-Driven Materials Discovery via Large Language Models (LLMs)
Harnessing the power of large language models, we are developing tools specifically designed for mining scientific literature and databases. Our goal is to extract critical insights and design principles hidden within vast amounts of unstructured data, enabling researchers to accelerate materials discovery and stay ahead in an increasingly data-driven world. In a notable project, we analyzed over 6 million research articles to identify promising materials for high-performance HEAs. Our models identified previously undiscovered compositions by assessing the contextual similarity of elements used in alloy development. This approach reduces the need for time-consuming literature reviews and opens new avenues for complex alloy designs.
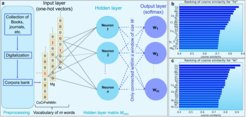
4. AI for Advanced Experimental Data Analysis
Characterizing materials at atomic and nanoscale resolution produces enormous datasets, often overwhelming traditional analysis methods. Our group focuses on developing AI frameworks that can analyze experimental data from Scanning Electron Microscopy (SEM), Transmission Electron Microscopy (TEM), Atom Probe Tomography (APT), and X-ray Diffraction (XRD). By automating and improving the precision of this analysis, we can extract meaningful insights from raw data faster and more reliably than ever before. One of our recent projects involves the application of a 3D convolutional neural network (CNN) model for direct recognition of crystal structures from APT data (Figure 3). This model achieves over 98% accuracy even with random displacements and missing atoms, advancing the identification of crystal structures in materials with high experimental noise. This work significantly enhances the precision of structure classification, particularly in complex alloys.
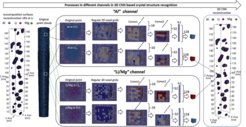
Why Join Us?
Our group is a hub for collaboration between AI researchers and materials scientists. We welcome students, postdocs, and researchers who are passionate about contributing to the next wave of breakthroughs in materials discovery and sustainable innovation. By joining us, you will:
- Work on highly interdisciplinary projects that push the limits of what AI and materials science can achieve together.
- Collaborate with world-class experts in AI, materials science, and experimental techniques.
- Contribute to cutting-edge research with real-world impact, from alloy design to the discovery of environmentally friendly materials.
- Gain experience with state-of-the-art machine learning models, high-performance computing, and advanced experimental setups.