The Spectral Nature of Solute-GB Interaction
Solute-grain boundary interaction can have a strong impact on material properties, even at very dilute solute concentrations. Traditionally this interaction is represented with a single segregation energy; in this project we use empirical potentials to demonstrate that using a spectral representation of the interaction is important for accurately capturing temperature dependent behavior. Furthermore, we use empirical potentials in combination with machine learning to efficiently determine these spectra.
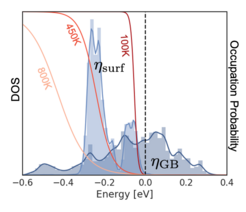
The segregation of solutes to grain boundaries (GBs) can play a very important role in the microstructural evolution and final material properties of a metal. This is true even when the alloying element is present at very low concentrations since GBs often act as powerful sinks for solutes, i.e., the segregation energies can be quite strong. Because this mechanism can play such an important role, solute-GB interactions have been the focus of many atomistic simulation studies. However, most studies – especially those using density functional theory, which severely limits system sizes – tend to focus on special high-symmetry boundaries.
In this project, we use empirical potentials to study solute segregation at a wide variety of low-symmetry aluminum GBs, which is representative of GBs found in nature. While high symmetry boundaries have only a small number of distinct segregation energies, at low symmetry boundaries there is a wide variety of local environments for the solute atoms to encounter. This leads to continuous spectra of interaction energies between the solute and the GB. With this in mind, we can apply tools that are useful for other spectra, e.g. exploiting the Fermi-Dirac occupation curve to determine the amount and location of segregated solute for a given temperature in a probabilistic way.
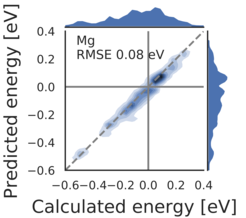
Even with empirical potentials, calculating these segregation spectra can be quite costly due to the sheer number of different local environments that might be encountered. To accelerate the process of producing these spectra, we have also demonstrated that each of the individual segregation energies can be captured to a high degree of accuracy with machine learned models. By building the model using structural data that is from near the segregation site and from the undecorated boundary, we are able to generate segregation from a single calculation instead of one calculation per segregation site.
Of course, GBs are not the only defect to which there is segregation. For instance, we have also begun to consider segregation to free surfaces, which also share this continuous spectral property for all but very special surface orientations. By comparing GB and free surface spectra, we can gain insight into the temperature dependence of a solute's embrittlement potency.