Big Data in Materials Research
Max-Planck-Institut für Eisenforschung combines big data and machine learning with atomic structure analysis
In the new initiative “MaxNet on Big-Data-Driven Materials Science” (short: BigMax) of the Max Planck Society, the Max-Planck-Institut für Eisenforschung (MPIE) cooperates with eleven other institutes of the Max Planck Society to explore how the recent successes in big data algorithms and machine learning can be employed to open new concepts in the discovery and design of advanced materials. The first funding period is five years. The MPIE concentrates on experimental data of atom probe tomography (APT) and field ion microscopy (FIM) on the one hand, and on the other hand its expertise in computational materials design.
“Current APT measurements detect up to 80% of the atoms in a sample. From this, only a fraction containing the highest data quality is used for further analysis, and that is very user dependent. There is no automated and systematic way to recognize certain patterns or compare different samples, which is made difficult by the shear size of the datasets that we obtain”, explains Dr. Baptiste Gault, leader of the APT group at the MPIE. As part of the BigMax initiative, his group has started a collaboration with the Max-Planck Computing and Data Facility in Garching to implement high-performance computing approaches to efficiently mine the APT data and automatically extract information. In addition, together with the MPIE’s department of Computational Materials Design the plan is to develop algorithms which boost the effective resolution of the APT experiments.
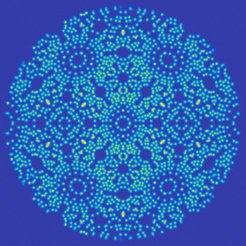
Field ion microscopy is comparable to APT but instead of ionizing individual atoms constituting the specimen’s tip, it ionizes gas atoms around the tip and detects this ionized gas on a phosphor screen. This way tens of thousands of images are taken which the scientists use to reconstruct a three-dimensional model of the atomic positions within the original specimen. Although APT has several advantages compared to FIM, such as providing direct compositional information, allowing to sample systems consisting of a billion atoms and being applicable even for alloys consisting of a large number of chemical species, presently only FIM allows to resolve atomic positions with a precision well beyond the atomic spacing. As such, FIM gives the unique opportunity to image important structures such as single atom vacancies directly. “APT doesn’t allow you to look directly at a vacancy but you rather see how the pattern around a probable vacancy is influenced. As only 80% of the atoms are detected, you cannot automatically conclude that an empty spot is a vacancy. Maybe it is an atom which was not detected at all”, says Shyam Katnagallu, PhD student in the APT group and working in close cooperation with the MPIE’s Computational Design Department. Although the FIM is a well-known method, the difficult data interpretation has progressively pushed it out of the spotlight. “We currently create algorithms to identify the same atomic structures in different FIM images even if some atoms are missing or shifted. We aim at achieving the same for APT at a later stage. Moreover, we implement the influence of the electrical field on the sample’s apparent geometry. I expect first promising results in two or three years”, clarifies Dr. Christoph Freysoldt, leader of the “Defect Chemistry and Spectroscopy” group.
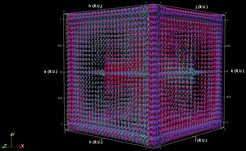
To boost the spatial and chemical resolution of the instrument, future algorithms will not only consider the entire specimen’s composition but all output collected by the instrument. As pointed out by Prof. Jörg Neugebauer, head of the department Computational Materials Design, “To successfully extract the relevant materials information in such a huge data space – a single measurement provides up to a billion data points –the use of advanced pattern matching and machine learning algorithms are required. Over the last years we have therefore developed an integrated development environment for computational materials science called pyiron that is capable to manage huge amounts of data and the complex simulation protocols efficiently and fast.” Parts of the reconstruction data gained by the MPIE will be given to the Fritz Haber Institute of the Max Planck Society to develop tools that will allow for predicting the mechanical behaviour based on atom probe data.
The aim of BigMax is to bundle the competence of twelve Max Planck institutes on big data algorithms, material science and computational simulations to identify new strategies how to use the huge amount of existing experimental and computational data to discover so far unknown relations in materials data space. The knowledge of these relations will enable new strategies to design materials. Examples will be structural materials with superior mechanical properties or thermoelectrics which allow to harvest electrical energy from waste heat. Besides the Max-Planck-Institut für Eisenforschung, the MPI for the Dynamics of Complex Technical Systems, of Colloids and Interfaces, of Microstructure Physics, for Polymer Research, for Biogeochemistry, for Physics of Complex Systems, for the Structure and Dynamics of Matter, for Intelligent Systems, for Informatics, the Max Planck Computing and Data Facility and the Fritz Haber Institute take part in this cooperation. The MPIE receives funding for two postdoctoral researchers, one for the experimental part of the APT and FIM measurements and one for the theoretical part. Furthermore, the MPIE plans to cooperate with research organizations outside the Max Planck Society and with industrial partners to foster Industry 4.0.
More information: https://www.bigmax.mpg.de/
Author: Yasmin Ahmed Salem, M.A.